eNews, Technology
How to ethically train AI
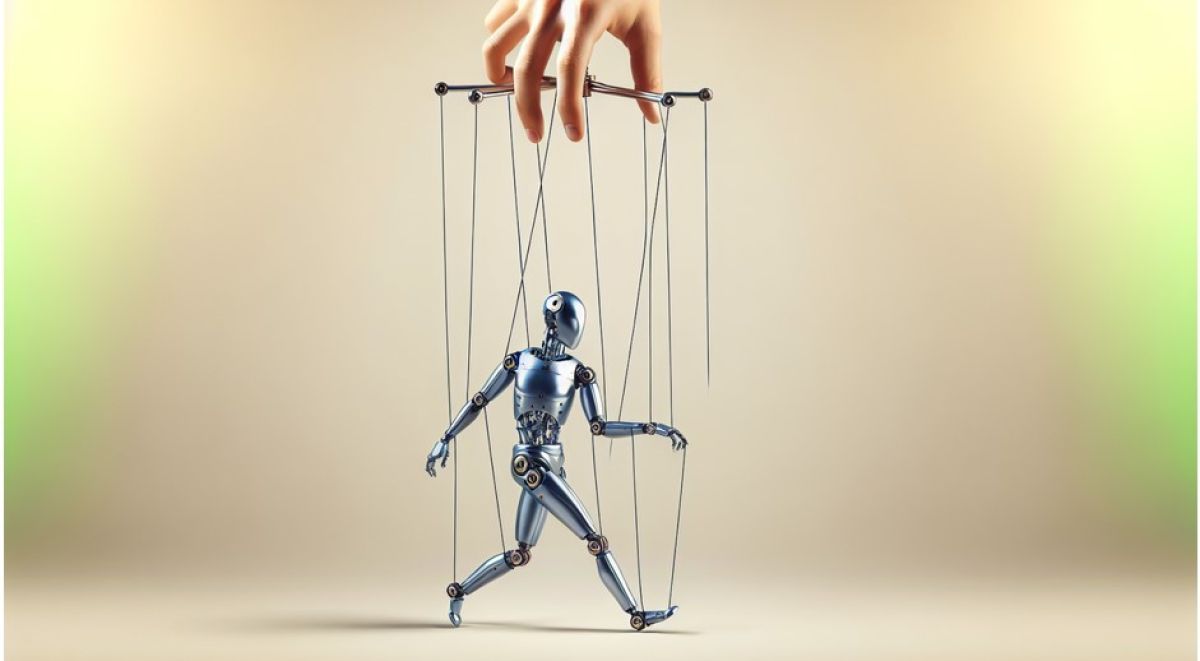
The rapid development of artificial intelligence has created a plethora of opportunities across the world, from aiding healthcare professionals with diagnoses to planning itineraries for vacation—or simplifying everyday tasks through automation processes.
Yes, but: The introduction to this tool has also raised many ethical concerns: Where does AI obtain its information or What are the sources of information for AI? How do we know it is being used correctly?
Why it matters: The truth is, all humans hold their own biases whether consciously or subconsciously—and because AI systems are created by humans, they can inherit the same bias. Our knowledge is based on predictive patterns through already-lived experience, thus, influencing AI outputs. So, how can we train AI ourselves to provide the most accurate information possible all while remaining ethical and unbiased?
First thing’s first: Here are some of the major do’s and don’ts when it comes to ethical AI use.
Do:
- Consider the risk environment.
- Notify others when AI is used to create or replace work processes.
- Take copyright implications into account.
- Always have a human double-check AI-generated work for any errors or inaccurate information.
Don’t:
- Assume 100% accuracy from AI-generated images, processes, suggestions, etc.
- Ignore biases in AI generated content.
- Prompt AI to suggest harmful or biased statements.
What they’re saying: “What science can’t put into AI is values and ethics,” said Martin Zorn, managing director of risk research and quantitative solutions at SAS Institute Inc. (Honolulu, HI). “We tend to already have bias on what we think the outcome should be, and that’s the biggest danger. When you set up those models, you are putting your biases into the model or the parametrization of the model.”
The building blocks of artificial intelligence can be broken down three different ways: Data selection, model selection and training and iteration.
- Your data selection becomes essential because not all data is considered “clean.” It is important to focus on how well you can clean that data before it is put into AI systems for use.
- Model selection focuses on what will analyze the data. This could be a robotic or manual process before inputting the information into AI.
- Training and iteration allow the machine to perform model selections so that when you ask AI a question, you get the best possible solution.
“We input a lot of data gleaned from public domain internet sites into our models,” said Zorn. “It may come from the Federal Reserve, the U.S. Treasury or the IMF. I could tell the AI [model], ‘Here’s our terms of use so alert me if any of our terms of use are compromised on any given day.’ And then that would protect us from missing information because it’s in the public domain.”
From a legal perspective: Lawyers mustguard against ethical lapses if they use generative artificial intelligence in their work, according to the American Bar Association. In its first formal ethics opinion on generative AI, an ABA committee said that any lawyer using AI must “fully consider their ethical obligations to protect clients,” including duties that fall under lawyer competence, confidentiality of client data, communication and fees.
Lawyers will typically use AI for tasks such as document drafting, document analysis or transactional necessities. There are also many new legal tech startups that are attracting investments as AI continues to develop. “Users must understand the full extent and limitation that AI’s results provide and the data being analyzed before drawing conclusions,” said Cecilia Cassella, CCE, manager, A/R at Glenmark Pharmaceuticals Inc. (Mahwah, NJ). “To the extent that certain steps can be automated, the design has to be clearly documented, tested and defined to avoid any misuse or misinterpretation of data.”
The most common uses of AI in credit management include:
- Payment trend analysis.
- Credit limit recommendations.
- Cash application process.
- Customer emails.
The bottom line: Ethics and regulations are essential to artificial intelligence processes. Any business using AI in their operations or part of their decision-making must ensure the AI program works in a fair and ethical manner.